With new technology comes new ways of communicating with one another in times of crisis. Platforms such as Twitter and Facebook allow important information to be shared widely and instantaneously.
But new technology is needed to extract and preserve the fruit of these new media, to allow crisis managers, crisis communicators and other key decision-makers to better manage a response.
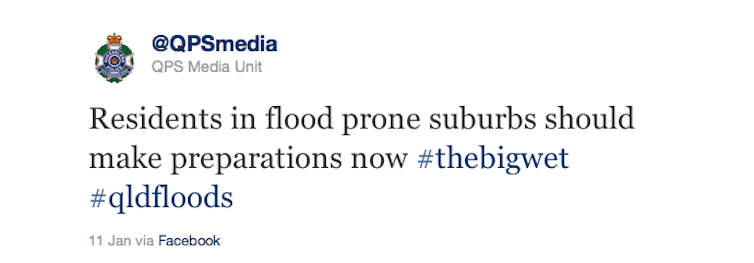
How can this type of information be ordered and made available to those who need it? Currently, the answer is “not very easily”. The hashtag, used on Twitter to share information on a common point of interest does some of this work, but is imperfect. Because anyone can use a given hashtag the information in the content stream is indiscriminate.
Crisis management leans on efficient and effective use of past experiences, experts’ tacit knowledge and information gathered on a given crisis.
The objective of my team at NICTA is to develop a search engine that captures information on humanitarian crises in social media.
So what will our search engine be able to do?
1) It will identify tweets that carry crisis information, using rule-based filtering techniques.
2) After this filtering phase, the remaining messages will be classified according to well-defined crisis management categories: “economy”, “food”, “environment”, “personal”, “community”, “political”, “health” and “unknown”.
3) It creates time trends of messages in each category, maps the messages to their geographic location, and identifies whether the author represents the government, media, or laypeople.
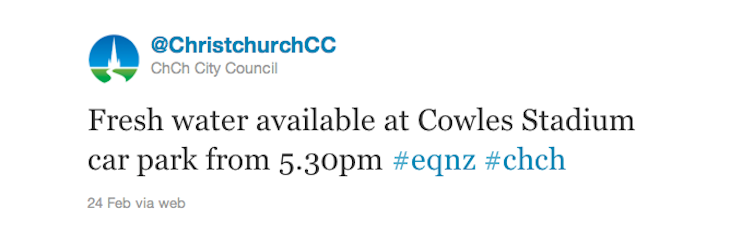
So, where is our search engine up to?
So far, we have introduced a conceptual model of our engine for information retrieval on Twitter from a human security viewpoint. The model pinpoints the added value of social media for crisis management and outlines real-life case studies for our technologies.
We have also defined the information search task. This includes both machine learning methods to implement the technologies and guidelines for gathering expert-annotated data for its validation.
This involved a crisis management expert in our team developing an annotation guideline and training two other team members to perform this manual classification task on a pilot data set of approximately 350 messages.
We have created two new data sets and their expert annotations.
The first data set includes over 50,000 Twitter messages and user profiles on the Christchurch earthquake in February of this year.
The second data set includes nearly 50,000 Twitter messages on the recent Queensland floods.
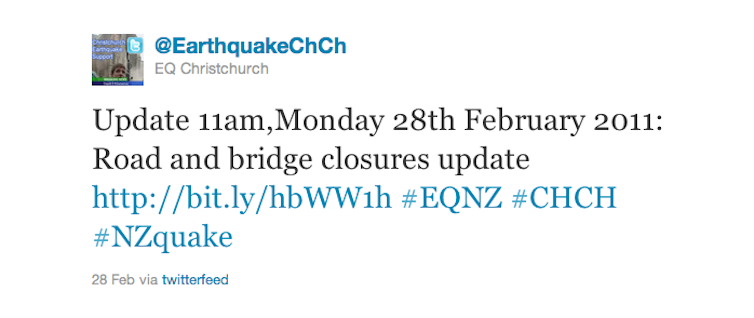
The expert annotations provided model solutions to our content classification task with categories of “economy”, “food”, “environment”, “personal”, “community”, “political”, “health” and “unknown”.
Then, the two team members who had been working with the crisis management expert annotated the final set of 1,000 messages. The high quality of our task definition, annotation guidelines and expert training was demonstrated by the team members’ almost perfect agreement in the classification task.
We have also seen positive early results on the automated task. We are currently improving our methods for identifying social media messages relating to our categories of “food”, “environment”, “personal”, “political” and “health” although the very limited number of relevant messages in our data sets is making the classification task more difficult.
On the category of “community”, the classification performance already looks promising. Finally, and most importantly, on the most prevalent category of “economic”, our performance is excellent, as is our ability to distinguish messages by government, media and laypeople.
Could this technology be used to great effect in future crisis management? We believe it could, and will keep working to ensure that happens.
For more information about computer-based decision support and Twitter, watch the recent video lectures by Aapo Immonen and Karl Kreiner.